Helen Toner is the Director of Strategy and Foundational Research Grants at Georgetown’s Center for Security and Emerging Technology (CSET). She previously worked as a Senior Research Analyst at Open Philanthropy, where she advised policymakers and grantmakers on AI policy and strategy. Between working at Open Philanthropy and joining CSET, Helen lived in Beijing, studying the Chinese AI ecosystem as a Research Affiliate of Oxford University’s Center for the Governance of AI. Helen has written for Foreign Affairs and other outlets on the national security implications of AI and machine learning for China and the United States, as well as testifying before the U.S.-China Economic and Security Review Commission. Helen holds an MA in Security Studies from Georgetown, as well as a BSc in Chemical Engineering and a Diploma in Languages from the University of Melbourne.
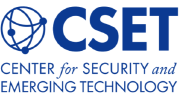

Directors,
Foundational Research Grants,
Strategy
Helen Toner
Director of Strategy and Foundational Research Grants Print BioRelated Content
Evaluating Large Language Models
July 2024Researchers, companies, and policymakers have dedicated increasing attention to evaluating large language models (LLMs). This explainer covers why researchers are interested in evaluations, as well as some common evaluations and associated challenges. While evaluations can… Read More
Key Concepts in AI Safety: Reliable Uncertainty Quantification in Machine Learning
June 2024This paper is the fifth installment in a series on “AI safety,” an area of machine learning research that aims to identify causes of unintended behavior in machine learning systems and develop tools to ensure… Read More
In an article published by Foreign Policy that discusses the recent bilateral meetings between China and the United States, CSET Director of Strategy and Foundational Research Grants, Helen Toner, provided her expert insights. Read More
CSET's Director of Strategy and Foundational Research Grants Helen Toner delivered a talk at TED2024 on the importance of developing smart AI policy, even in the face of uncertainty. Read More
In their op-ed featured in Lawfare, CSET’s Matthew Burtell and Helen Toner shared their expert analysis on the significant implications of government procurement and deployment of artificial intelligence (AI) systems, emphasizing the need for high… Read More
How to govern AI in the face of uncertainty?
March 2024In EqualAI's podcast 'In AI We Trust?', Helen Toner discusses key AI issues like China's policies, AI in warfare, and regulation challenges. Read More
Large language models (LLMs), the technology that powers generative artificial intelligence (AI) products like ChatGPT or Google Gemini, are often thought of as chatbots that predict the next word. But that isn't the full story… Read More
Large language models (LLMs), the technology that powers generative artificial intelligence (AI) products like ChatGPT or Google Gemini, are often thought of as chatbots that predict the next word. But that isn't the full story… Read More
Large language models (LLMs), the technology that powers generative artificial intelligence (AI) products like ChatGPT or Google Gemini, are often thought of as chatbots that predict the next word. But that isn't the full story… Read More
In an Australian Broadcasting Corporation 7.30 segment that discusses the concerns about the current understanding of AI technology, Helen Toner provided her expert insights. Read More
CSET's Helen Toner provided her expert insights with Bloomberg about the competition between the United States and China in the development of artificial intelligence (AI) technology. Read More
Recent advances in general-purpose artificial intelligence systems have sparked interest in where the frontier of the field might move next—and what policymakers can do to manage emerging risks. This blog post summarizes key takeaways from… Read More
AI capabilities are evolving quickly and pose novel—and likely significant—risks. In these rapidly changing conditions, how can policymakers effectively anticipate and manage risks from the most advanced and capable AI systems at the frontier of… Read More
How can policymakers credibly reveal and assess intentions in the field of artificial intelligence? Policymakers can send credible signals of their intent by making pledges or committing to undertaking certain actions for which they will… Read More
This data brief uses procurement records published by the U.S. Department of Defense and China’s People’s Liberation Army between April and November of 2020 to assess, and, where appropriate, compare what each military is buying… Read More
How can we measure the reliability of machine learning systems? And do these measures really help us predict real world performance? A recent study by the Stanford Intelligent Systems Laboratory, supported by CSET funding, provides… Read More
During her interview with ABC News Live, CSET's Helen Toner delved into the significant growth of Artificial Intelligence, with a particular emphasis on its impact within the realm of National Security. Read More
In an op-ed published in TIME, CSET's Helen Toner discusses the challenges of understanding and interacting with chatbots powered by large language models, a form of artificial intelligence. … Read More
What exactly are the differences between generative AI, large language models, and foundation models? This post aims to clarify what each of these three terms mean, how they overlap, and how they differ. Read More
Will China Set Global Tech Standards?
March 2022Director of Strategy Helen Toner explored China's tech standards in China File. Read More
Problems of AI safety are the subject of increasing interest for engineers and policymakers alike. This brief uses the CSET Map of Science to investigate how research into three areas of AI safety — robustness,… Read More
Privacy is Power
January 2022In an opinion piece for Foreign Affairs, a team of CSET authors alongside coauthors make the case for the use of privacy-enhancing technologies to protect personal privacy. Read More
This paper is the fourth installment in a series on “AI safety,” an area of machine learning research that aims to identify causes of unintended behavior in machine learning systems and develop tools to ensure… Read More
‘Small Data’ Is Also Crucial for Machine Learning
October 2021In their op-ed for Scientific American, Husanjot Chahal and Helen Toner argue how small data can assist AI breakthroughs. Read More
Conventional wisdom suggests that cutting-edge artificial intelligence is dependent on large volumes of data. An overemphasis on “big data” ignores the existence—and underestimates the potential—of several AI approaches that do not require massive labeled datasets. Read More
As modern machine learning systems become more widely used, the potential costs of malfunctions grow. This policy brief describes how trends we already see today—both in newly deployed artificial intelligence systems and in older technologies—show… Read More
This paper is the third installment in a series on “AI safety,” an area of machine learning research that aims to identify causes of unintended behavior in machine learning systems and develop tools to ensure… Read More
This paper is the second installment in a series on “AI safety,” an area of machine learning research that aims to identify causes of unintended behavior in machine learning systems and develop tools to ensure… Read More
This paper is the first installment in a series on “AI safety,” an area of machine learning research that aims to identify causes of unintended behavior in machine learning systems and develop tools to ensure… Read More
Why robustness is key to deploying AI
June 2020"When it comes to high-stakes settings," write Jacob Steinhardt and CSET's Helen Toner, "machine learning is a risky choice." Their piece in Brookings TechStream offers rules of thumb for assessing risk of failure in ML. Read More
CSET's Helen Toner highlights OpenAI's delayed release of GPT-2 and the increased attention it brought to publication norms in the AI research community in 2019. This piece was featured in In the Shanghai Institute for… Read More
CSET’s Helen Toner, Jeff Ding, and Elsa Kania testified before the U.S.-China Economic and Security Review Commission on U.S.-China Competition in Artificial Intelligence: Policy, Industry, and Strategy. Read More
Helen Toner’s Testimony Before the U.S.-China Economic and Security Review Commission
June 2019CSET Director of Strategy Helen Toner testified before the U.S.-China Economic and Security Review Commission at a hearing on "Technology, Trade, and Military-Civil Fusion: China’s Pursuit of Artificial Intelligence, New Materials, and New Energy."… Read More