In recent years, machine learning systems have been applied to an ever greater number of domains. This has been accompanied by a large tech industry push to adopt regulation that would allow these technologies to be applied to safety-critical applications, for example in medical decision making, the civilian aviation and automotive sectors, or financial applications. In such safety-critical areas, great care must be taken to appropriately assess the safety and robustness properties of these systems, often before tests in real-world scenarios are possible.
Summary of approach and findings
Building robust, reliable machine learning systems is notoriously difficult. Policymakers and engineers alike need to decide how to evaluate the robustness of a given system, often without having access to real-world evaluations. This presents a key question: How can we choose and design metrics for reliability such that achieving good results “in the lab” will give us confidence that the model will perform well in practice? And further, do good results on specific metrics imply a general understanding of the data, such that we can assume good results on other (possibly unmeasured) metrics as well?
To shine light on these questions, in this project we investigated five different metrics researchers commonly use to assess the reliability of machine learning models, and studied the relationships between these metrics across a variety of datasets, models, and training strategies. To do this, we evaluated more than 500 models on three different computer vision tasks, namely (i) animal species recognition, (ii) cancer classification in medical images, and (iii) land-use classification from satellite images (see Figure 1).
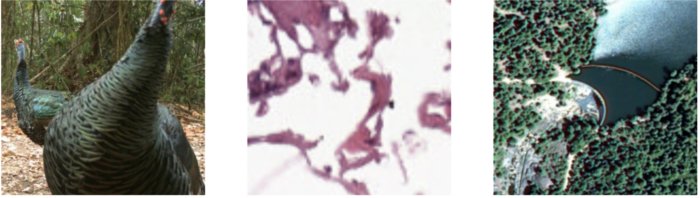
By assessing the performance of a wide range of models and training strategies applied to these different tasks, we could analyze how different reliability metrics relate to each other – for example, whether an increase in prediction accuracy also correlates with an increase in accuracy for similar data distributions, or an improved rate of detecting outliers. What we found, however, was that many training or model adjustments that increase performance in one reliability metric do not increase the overall “model capability” or “robustness” in a more holistic sense across multiple metrics. We note that similar results have been presented in recent work by Google Research.
However, we also find that several techniques improve performance over multiple metrics, indicating that they may improve the model on a more fundamental level. In particular, we find that instead of training new models from scratch, one particularly promising approach was to start with pre-trained models, then finetune them to the task at hand. Further, pre-training across multiple data modalities, such as images paired with natural language, produced the best results.
Our findings have important implications for policymakers and standards-setting bodies currently working to nail down requirements to apply to machine learning in high-risk or safety-critical areas. We cannot assume that machine learning models that perform well on one type of reliability metric will perform reliably in a broader sense. This means that we need to be careful in choosing how to turn the plain language idea of “reliability” into specific, technical metrics.
How to measure reliability
Measuring reliability is challenging, because it’s not clear what exactly “reliability” entails. For example, in its AI Risk Management Framework, the National Institute of Standards and Technology (NIST) defines reliability as the “ability of an item to perform as required, without failure, for a given time interval, under given conditions” and further relates this to robustness or generalization, defined as the “ability of an AI system to maintain its level of performance under a variety of circumstances.” This provides a rough definition, but leaves many questions open—what does it mean to “perform as required”? And what “variety of circumstances” are relevant? Building reliable machine learning systems requires us to convert this kind of plain language into specific technical requirements.
In order to conduct concrete measurements of a model’s reliability, we established a holistic view of a model’s performance in different circumstances by proposing a set of five “holistic reliability metrics” that span a minimum number of facets related to reliability:
- Performance on data similar to data used for training (“in-distribution”),
- Performance on data mostly similar to data used during training, but with some variation (“near-distribution”),
- Ability to identify data that is significantly different from data used during training (“out-of-distribution”),
- Quality of estimating prediction confidence (“uncertainty calibration”), and
- Robustness to adversarially altered inputs (“adversarial robustness”).
To illustrate these five metrics on an example, consider a medical image classification model trained to recognize tumors. In-distribution performance may refer to the prediction accuracy on images from the same imaging sensor as used during training, while images taken by sensors from different hospitals or sensor manufacturers would be examples of near-distribution data. Out-of-distribution examples could arise for new or unseen types of tumor. In this case, the model would ideally recognize it cannot perform well and flag the image as “out-of-distribution.” Uncertainty calibration relates to how well the confidence of the model’s predictions correspond to actual observations. For instance, across all images that the model predicts have a less than 1% chance of containing a tumor, we would want less than 1% of those samples to actually contain a tumor. Finally, adversarial robustness refers to robustness to an adversary “tampering” with the image, trying to fool the AI model. Although typically less applicable in medical imaging, this is important in applications like content moderation.
Ideally, a model should perform well on all of these metrics, though the importance of different metrics may vary for different use cases.
Improving one metric may not improve others
Research on machine learning reliability often only considers one or two of these metrics. Here, we investigate how the different metrics relate to each other in order to evaluate key questions like: is it actually necessary to test for all of them, or does good performance on one metric correspond to good performance across the board?
For instance, if we design two different metrics for different types of distribution shifts (“near-distribution” and “out-of-distribution”), we might expect that a model that performs well on one metric also performs well on the other and that an intervention that improves the first also improves the second. The hope would be that by optimizing for one metric, we can actually optimize more fundamental properties of the model, ideally improving the model’s overall “understanding” of the inputs (rather than just its performance on one metric). The ultimate goal is for improvements in properties we measure to correspond to improvements in properties we can’t fully measure, such as real-world performance.
In order to further understand these relationships, we explored a variety of training modifications to improve model performance. These approaches (which we describe in full in our paper) included modifications to multiple different stages of the process of training models, such as the selection of training data, model architecture, and training procedure. Interestingly, we found that almost all of these modifications improve only one metric at a time, with little correlation between metrics (see Figure 2). For example, augmenting the dataset (i.e. adding multiple slightly edited versions of images to increase the amount of training data) does affect near-distribution robustness, but provides no clear improvement on uncertainty calibration or out-of-distribution robustness. Similarly, methods aimed at improving uncertainty calibration generally don’t improve other metrics.
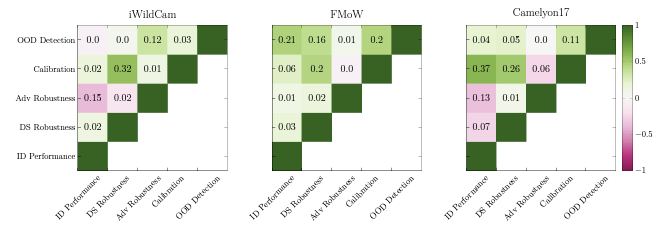
We can draw two conclusions from this:
- For unrelated metrics, it seems possible to devise training modifications that improve one metric without causing damage to others. This suggests that, given a “complete” set of metrics, we could find a set of training modifications that each improve one metric at a time, resulting in overall improvements in all metrics.
- This also suggests that any unmeasured metrics—for example those representing real-world performance—might be unaffected by our measured metric improvements.
Pre-training to the rescue?
Although most training modifications we investigated only improved on metric at a time, we did find a number of modifications that uniformly improved our metrics. The most promising approach is called pre-training. When training a model, a practitioner may choose to either start from scratch with an uninitialized model (i.e. an approximately random starting point), or start with a model that is already “pre-trained” to a vaguely related task. For instance, one can pre-train a model for an image-related task by collecting a set of natural images, manually obfuscating patches of the image, and then training the model to recover these patches. After this pre-training process, models can then be further “fine-tuned” to perform well on more specific tasks. Figure 3 depicts how choosing such a pre-trained model as the base model improved all of our reliability metrics, at the cost of a small reduction in in-distribution performance. Specifically, we find that leveraging pre-training can outperform the randomly initialized models (dashed baseline) by over 10% in our proposed holistic reliability score, and conclude that this performance improvement is mainly due to an improvement in robustness.
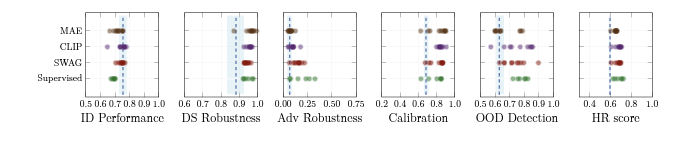
Pre-training has also been successfully applied to many other machine learning tasks, and has led to some well-known recent advances in AI. For instance, large language models such as OpenAI’s ChatGPT (which stands for “Generative Pre-trained Transformer”) are typically developed by first pre-training a model to predict the next word based on a huge corpus of text data, before being fine-tuned to more specific language-based tasks (such as being a chatbot).
The usage of pre-trained models, however, also comes with some notable downsides. Practitioners often build on models that were pre-trained by others. This means that unless details about the full training procedure are provided, there may be concerns about the source and quality of data used, including implications for data privacy, implicit biases, and intellectual property rights. This comes atop the possibility of security flaws (e.g. backdoors/”Trojans”) which can be accidentally or purposefully included in the model, and may lead to data leaks or adversarial backdoors.
Policy Implications
From a policy perspective, the primary implication of our findings is that we should not think of “reliability” or “robustness” as a single, easy-to-measure property of an AI system. Rather, a system that is reliable in one sense (e.g. being able to adapt from one operating environment to another) may not be reliable in another (e.g. being able to withstand adversarial attempts to spoof it).
When creating regulatory definitions or standards for AI—such as during the standardization process soon to be kicked off by the European Union’s AI Act—policymakers will have to grapple with this challenge. Attempting to create a single technical specification or testing procedure for reliability would be mistaken. Instead, AI reliability requirements will need to consider which facets of reliability matter most for any given use case, and how those facets can be tested for.
Our results suggest that further advances in AI, perhaps taking into account an even greater variety of “pre-training” data, may make it more straightforward to develop reliable AI systems. However, it is still unclear which failure modes can and can not be fixed by such a strategy; and new failure modes may yet to be uncovered.
Finally, we conclude that in spite of the many questions that remain, there is already a great variety of tools and metrics applicable to current systems. We are therefore looking forward to upcoming policy developments that will provide guidance for both researchers and practitioners in closing the gap to developing robust and reliable systems.
This research project, conducted by the Stanford Intelligent Systems Laboratory, was funded by Foundational Research Grants, a CSET program that supports technical research to shed light on the potential national security implications of AI over the long term. This blog post summarizes the findings and implications of the Stanford team’s work, which are described in more detail in a technical paper.