Andrew Lohn is a Senior Fellow at Georgetown’s Center for Security and Emerging Technology (CSET). He previously served as the Director for Emerging Technology on the National Security Council Staff, Executive Office of the President, under an Interdepartmental Personnel Act agreement with CSET. Prior to joining CSET, he was an Information Scientist at the RAND Corporation, where he led research focusing mainly on cybersecurity and artificial intelligence. Prior to RAND, Andrew worked in material science and nanotechnology at Sandia National Laboratories, NASA, Hewlett Packard Labs, and a few startup companies. He has published in a variety of fields and his work has been covered in MIT Technology Review, Gizmodo, Foreign Policy and BBC. He has a PhD in electrical engineering from UC Santa Cruz and a Bachelors in Engineering from McMaster University.
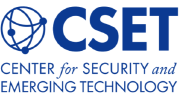
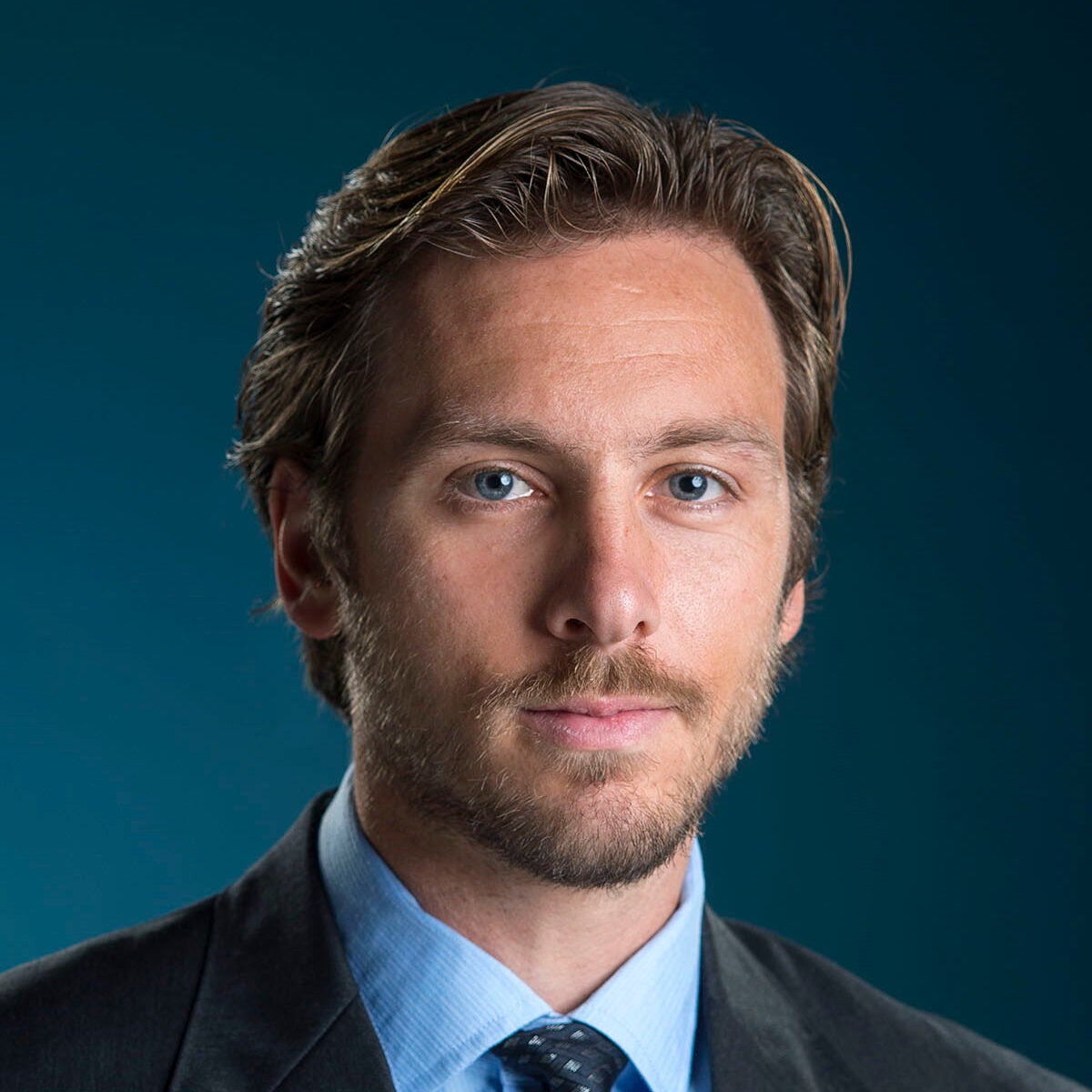
Analysis,
CyberAI Project
Andrew Lohn
Executive Office of the President, National Security Council Staff Print BioRelated Content
In an article published by the Brennan Center for Justice, Josh A. Goldstein and Andrew Lohn delve into the concerns about the spread of misleading deepfakes and the liar's dividend. Read More
While recent progress in artificial intelligence (AI) has relied primarily on increasing the size and scale of the models and computing budgets for training, we ask if those trends will continue. Financial incentives are against… Read More
Concerns over risks from generative artificial intelligence systems have increased significantly over the past year, driven in large part by the advent of increasingly capable large language models. But, how do AI developers attempt to… Read More
This explainer overviews techniques to produce smaller and more efficient language models that require fewer resources to develop and operate. Importantly, information on how to leverage these techniques, and many of the subsequent small models,… Read More
Artificial intelligence that makes news headlines, such as ChatGPT, typically runs in well-maintained data centers with an abundant supply of compute and power. However, these resources are more limited on many systems in the real… Read More
Two CSET researchers are coauthors for a new multi-organization report about the safety of AI systems led by OpenAI and the Berkeley Risk and Security Lab. The report, published on arXiv, identified six confidence-building measures… Read More
Securing AI Makes for Safer AI
July 2023Recent discussions of AI have focused on safety, reliability, and other risks. Lost in this debate is the real need to secure AI against malicious actors. This blog post applies lessons from traditional cybersecurity to… Read More
The current AI-for-cybersecurity paradigm focuses on detection using automated tools, but it has largely neglected holistic autonomous cyber defense systems — ones that can act without human tasking. That is poised to change as tools… Read More
CSET's Andrew Lohn and Joshua A. Goldstein share their insights on the difficulties of identifying AI-generated text in disinformation campaigns in their op-ed in Lawfare. Read More
Progress in artificial intelligence (AI) depends on talented researchers, well-designed algorithms, quality datasets, and powerful hardware. The relative importance of these factors is often debated, with many recent “notable” models requiring massive expenditures of advanced… Read More
Artificial intelligence systems are rapidly being deployed in all sectors of the economy, yet significant research has demonstrated that these systems can be vulnerable to a wide array of attacks. How different are these problems… Read More
Funding and priorities for technology development today determine the terrain for digital battles tomorrow, and they provide the arsenals for both attackers and defenders. Unfortunately, researchers and strategists disagree on which technologies will ultimately be… Read More
Andrew Lohn’s Testimony Before the House Homeland Security Subcommittee on Cybersecurity, Infrastructure Protection, and Innovation
June 2022CSET Senior Fellow Andrew Lohn testified before the House of Representatives Homeland Security Subcommittee on Cybersecurity, Infrastructure Protection, and Innovation at a hearing on "Securing the Future: Harnessing the Potential of Emerging Technologies While Mitigating… Read More
Andrew Lohn’s Testimony before House of Representatives Science, Space and Technology Subcommittees
May 2022CSET Senior Fellow Andrew Lohn testified before the House of Representatives Science, Space and Technology Subcommittee on Investigations and Oversight and Subcommittee on Research and Technology at a hearing on "Securing the Digital Commons: Open-Source… Read More
Andrew Lohn’s Testimony before the Senate Armed Services Subcommittee on Cybersecurity
May 2022CSET Senior Fellow Andrew Lohn testified before the U.S. Senate Armed Services Subcommittee on Cybersecurity hearing on artificial intelligence applications to operations in cyberspace. Lohn discussed AI's capabilities and vulnerabilities in cyber defenses and offenses. Read More
Like traditional software, vulnerabilities in machine learning software can lead to sabotage or information leakages. Also like traditional software, sharing information about vulnerabilities helps defenders protect their systems and helps attackers exploit them. This brief… Read More
Between 2012 and 2018, the amount of computing power used by record-breaking artificial intelligence models doubled every 3.4 months. Even with money pouring into the AI field, this trendline is unsustainable. Because of cost, hardware… Read More
Modern machine learning often relies on open-source datasets, pretrained models, and machine learning libraries from across the internet, but are those resources safe to use? Previously successful digital supply chain attacks against cyber infrastructure suggest… Read More
Growing popular and industry interest in high-performing natural language generation models has led to concerns that such models could be used to generate automated disinformation at scale. This report examines the capabilities of GPT-3--a cutting-edge… Read More
Machine learning systems’ vulnerabilities are pervasive. Hackers and adversaries can easily exploit them. As such, managing the risks is too large a task for the technology community to handle alone. In this primer, Andrew Lohn… Read More