Executive Summary
Automation bias is the tendency for an individual to over-rely on an automated system. It can lead to increased risk of accidents, errors, and other adverse outcomes when individuals and organizations favor the output or suggestion of the system, even in the face of contradictory information.
Automation bias can endanger the successful use of artificial intelligence by eroding the user’s ability to meaningfully control an AI system. As AI systems have proliferated, so too have incidents where these systems have failed or erred in various ways, and human users have failed to correct or recognize these behaviors.
This study provides a three-tiered framework to understand automation bias by examining the role of users, technical design, and organizations in influencing automation bias. It presents case studies on each of these factors, then offers lessons learned and corresponding recommendations.
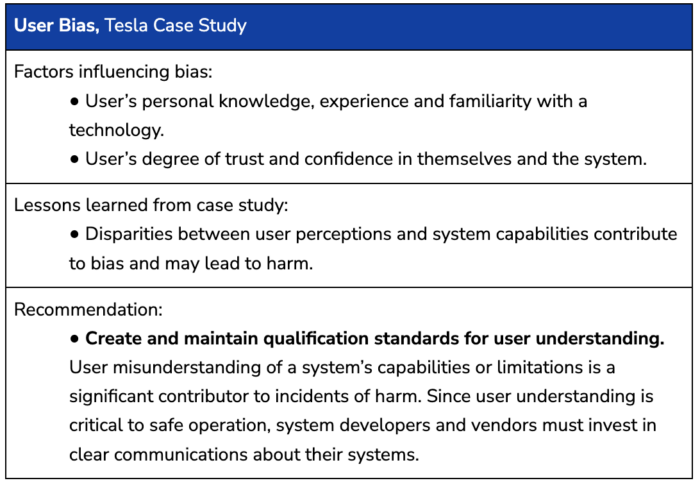

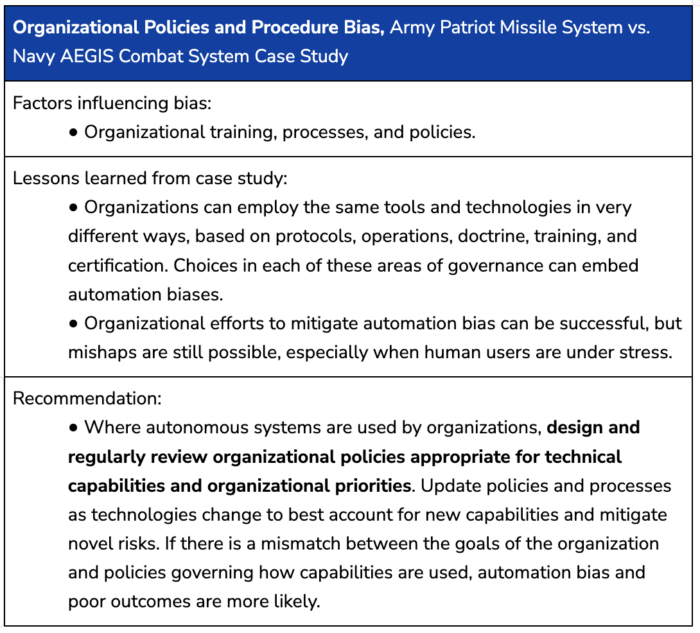
Across these three case studies, it is clear that “human-in-the-loop” cannot prevent all accidents or errors. Properly calibrating technical and human fail-safes for AI, however, poses the best chance for mitigating the risks of using AI systems.