As technology competition intensifies between the United States and China, governments and policy researchers are looking for metrics to assess each country’s relative strengths and weaknesses. One measure of technology innovation increasingly used by the policy community is research output. To inform this area of policy research, CSET maintains a merged corpus of scholarly literature and has published a range of analyses using it, as well as the Map of Science and the Country Activity Tracker (CAT), two public, interactive tools for exploring the global research landscape. Drawing on CSET’s experiences developing these tools over the last four years and using them for policy analysis, we share our best practices for using research output to study national technological competition.
Key Takeaways
- Assessments of national research output can vary widely depending on the data and methods used.
- Research output is not the same as innovation or technology leadership, and research output alone is not indicative of innovation capacity or technology leadership standing.
- Because academic research is both competitive and cooperative, it is important to account for collaborative work and co-authorship when examining research output.
- Transparency about sources and methods is paramount, as differences in data can yield different results. This is especially critical when dealing with emerging technology areas that are not yet established and often hard to define.
- Assessing national standing in emerging technology areas is complex. As such, findings should be framed carefully and cautiously, with key uncertainties flagged and appropriate caveats provided throughout the analysis.
Best Practices
Public research output is not the same as innovation or technology leadership. Research is one input to innovation, but counting research papers does not capture the full picture of technology development or leadership. This is especially true in domains dominated by militaries or commercial actors, where publication is less common. Because published research represents only one aspect of innovation, at CSET we evaluate additional metrics, such as patents, investment, company capabilities and market shares, and governance, when drawing overall conclusions about national competitiveness in science and technology (see CAT as well as prior CSET analysis here and here; see also here).1
Box 1. Update on CSET analysis of collaborative research output A 2021 CSET report found that between 2010-2019 scientific papers with international collaboration were cited more than non-collaborative papers. The gap varied by country, but was largest when comparing China’s collaborative and non-collaborative research. Updating this analysis through 2022, this result holds; While 33 percent of China’s collaborative publications are highly cited, only 10 percent of its non-collaborative ones are highly cited (Figure 1).2 Figure 1: Percentage of highly cited (90th citation percentile) collaborative and non-collaborative publications in all fields by country, 2010-2022. Source: CSET merged corpus We updated another 2021 analysis that compared U.S. and Chinese contributions to highly cited AI research. Using CSET’s research clusters, we found that the share of each country’s contributions to highly cited AI research varied based on the threshold for highly cited research, and that a notable share of both country’s contributions were U.S.-Chinese collaborations. We update that analysis using our AI classifier to find relevant research and find that the United States produced a greater share of AI research above the 90th citation percentile than China from 2010 to 2015, but that China’s share exceeded the United States’ share in 2016 (Figure 2). We still find a notable proportion of this research is U.S.-China collaboration. In 2021, 28 percent of the U.S.-affiliated papers and about 17 percent of China-affiliated highly cited AI papers were U.S.-China collaborations. Without considering these collaborative ties, a comparison of U.S. and China’s research output would be incomplete. Figure 2: Share of highly cited AI papers (90th citation percentile) affiliated with the United States and China, 2010-2021. Source: CSET merged corpus To further contextualize the rise in China’s AI highly cited research output, we separate research with international collaboration. We see much of China’s increase in highly cited AI research relative to the U.S. was from non-collaborative research (Figure 3). Figure 3: Number of highly cited AI papers (90th citation percentile) affiliated with the United States and China by collaboration type, 2010-2021. Source: CSET merged corpus |
Determinations of research leadership depend on methodological decisions. Research output is a measure of research leadership, but decisions about how to measure output influence who we see as leading. Previous CSET research found that looking at different levels of aggregation (e.g. a broad scientific discipline like computer science versus a specific field like machine learning) can lead to different conclusions in terms of what country is the top research producer in an area. Another CSET analysis found that U.S. and Chinese highly cited AI research output varies based on the threshold for “highly cited” research. The method used to assign papers to countries will also result in different paper counts, which could impact determinations of research leadership. When a paper has multiple authors, we don’t directly observe how much each coauthor contributed to the paper or research, so at CSET we assign full credit to each country with an author-affiliated research institution; a paper with one author affiliated with a Chinese institution and one author affiliated with a U.S. institution is added as one to each country’s paper count (see Table 1).
Table 1: Methods for counting publication authorship at the country level.
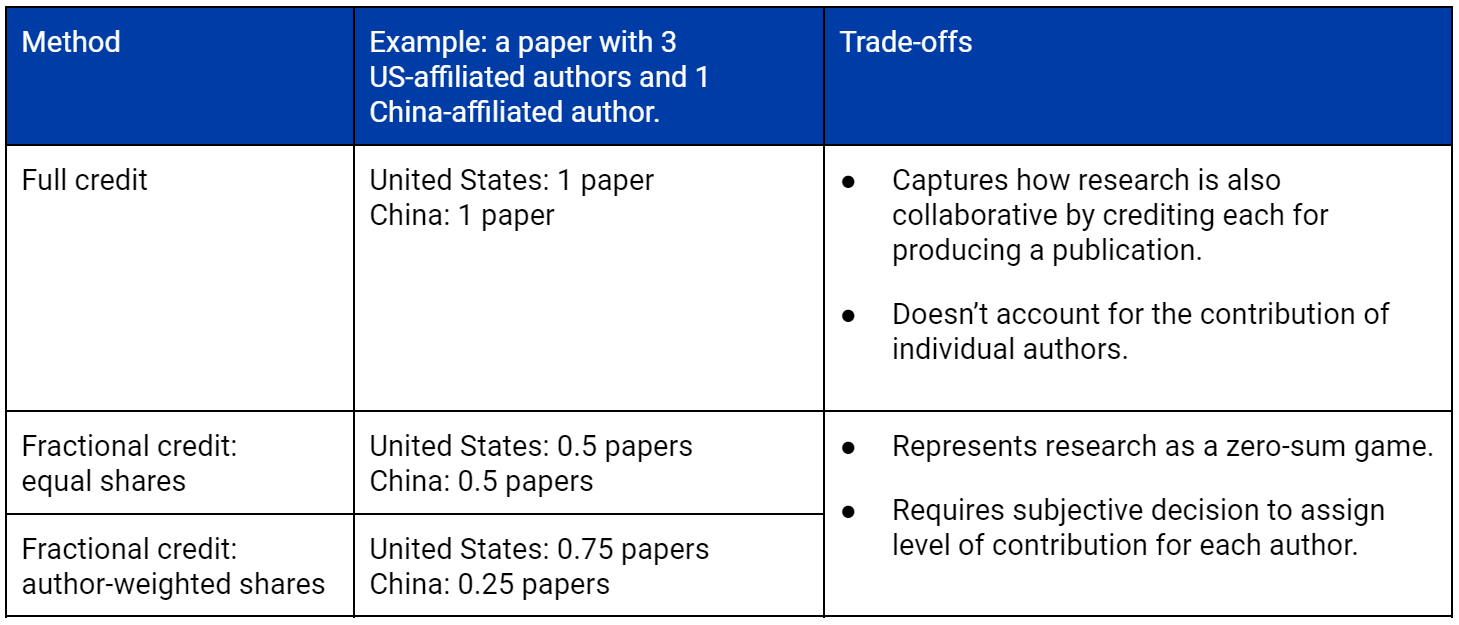
The corpus of research under study matters. We can get different results when we analyze different data. This means findings from any single analysis are limited and should be open to revision and updates. It is thus important to be transparent about sources, and why decisions about the sources used were made.3 CSET analysis of research relies on a corpus that combines and deduplicates publications from six different sources, including one which provides Chinese language research, which means our findings can differ from analyses relying on single sources (see Counting AI Research for more discussion on this point).
The method for identifying relevant research also matters. By their nature, emerging technology areas are not established and hard to define (see Through a Glass, Darkly: Mapping Emerging Technology Supply Chains Using CSET’s Map of Science and Supply Chain Explorer, forthcoming), and how we choose to define them matters for the conclusions we draw. There are several approaches to finding research relevant to a topic of interest. At CSET, we use a trained classifier to find AI-relevant research, and rely on predicted fields of study for other subjects. We also clustered our corpus based on citations, resulting in research clusters that often correspond to research areas of interest. We sometimes use keyword searches to identify relevant tech areas, but there are limitations to keyword-based research retrieval methods – most notably challenges related to maintaining, updating, and replicating those searches. CSET’s best practice is to systematically evaluate the results of keyword searches against known “ground truth” corpora before deploying them in our analysis (see Identifying AI Research, forthcoming, for more discussion on this point).
Box 2. Trends in national AI research output: how different sources and methods shape the analysis To illustrate how results can vary depending on sources and methods, we compare U.S. and Chinese research output in machine learning and natural language processing—topics also covered in a recent report by the Australian Strategic Policy Institute. We applied similar methods to those used by ASPI to run the analysis on our data. There are four changes between our analysis and ASPI’s.4 First, we used CSET’s merged research corpus as our source of research papers, whereas ASPI used Web of Science.5 Second, we used CSET’s field of study classification to find articles relevant to machine learning and natural language processing, while ASPI used keyword searches over article titles and abstracts. Third, CSET counts authorship using full credit while ASPI counts using author-weighted fractional credit (Table 1). Fourth, ASPI calculated their citation percentiles for the set of papers included in their emerging technology areas, identified by keyword search, while CSET calculated citation percentiles based on subject fields. Comparing our results to those reported by ASPI, we find similarities but also notable differences. We find that from 2018 to 2022, Chinese-affiliated researchers published more highly cited AI research (above the 90th percentile) than U.S. researchers (Figure 2). But looking at specific subfields, we find U.S. affiliated researchers produced more highly cited machine learning and natural language processing research. ASPI’s analysis found that Chinese-affiliated researchers produced more highly cited machine learning research than U.S. researchers and both produced comparable shares of highly cited natural language processing research. This shows that differences in the corpus being analyzed, the method used to find relevant research, and the method for assigning papers to countries can result in different answers to the question of “who is leading in this research area?” Table 2: Comparing Results Across Corpora and Classification Method (90th citation percentile), 2018-2022. Source: CSET merged corpus and ASPI Critical Technology Tracker |
Caveat results appropriately and throughout the presentation of results. Given the above considerations, a final best practice is to be clear about limitations. A primary one tends to stem from the representativeness of the data used; what we observe, collect, and analyze is almost always a sample of a larger whole, whether papers or people. Presentation of analytic results should discuss the possible effect of data collection and how findings are bound to the sample analyzed. At a higher level, this means avoiding conclusions that generalize beyond what was studied and not sensationalizing findings or framing them as definitive. At CSET, we are careful to present our findings as subject to context and nuance, and we encourage others to supplement our results with additional research. In practice, this means we do not consider our analyses of comparative national standing in AI research as a singular indicator of who is winning an “AI race,” but as one piece of evidence to inform a much larger discussion around national competition and cooperation in technological development.
- ETO’s CAT tracks global artificial intelligence (AI) research, patent, and investment activity by country or region, with detailed documentation outlining methodology, data sources, and suggested use. Specific to research, CAT provides several metrics including the number of AI papers published, but also the number of citations of those papers, the number and share of papers that are international collaborations, and change in output over time.
- The original analysis filtered by specific document types. For consistency with the other analyses in boxes 1 and 2, we removed that filter. Note that for all CSET analyses in this post, we exclude any publications without a single linkage (i.e. every publication in our corpus must cite another publication or be cited by another publication)
- In extreme situations, concerns about burning sources of information and vendor restrictions can justify withholding details on our data collection methodology. But these concerns should rarely prevent us from describing our analytic methods.
- Like ASPI, we filtered to publications in the 90th citation percentile or above that were published from 2018-2022 inclusive.
- CSET’s merged corpus of scholarly literature combines and de-duplicates publications from Digital Science Dimensions, Clarivate’s Web of Science, Microsoft Academic Graph, China National Knowledge Infrastructure, arXiv, and Papers With Code. Data sourced from Dimensions, an inter-linked research information system provided by Digital Science (http://www.dimensions.ai). All China National Knowledge Infrastructure content is furnished for use in the United States by East View Information Services, Minneapolis, MN, USA.