AlphaFold is a novel technology that uses artificial intelligence to predict the three-dimensional shape of an individual protein based on its atomic sequence and DNA. Previously, it could take months and significant labor from scientists to find the 3-D structure of an individual protein. Now, because of this experimental data, an enormous databank of 3-D protein structures is available to scientists worldwide, several decades earlier than expected. The latest iteration of AlphaFold’s source code was released by Google’s DeepMind in July 2021. This latest release will have implications for bioinformatics, medicine, and science that relies on genetic data.
AlphaFold is the culmination of several decades of biological and computational research. The fields of biological research that helped drive the development of AlphaFold include deep mutational scanning (DMS) and protein structure prediction (PSP), the latter of which is AlphaFold’s function, partially resolving the protein folding problem (PFP).
DMS employs sequencing technologies to measure possible variants of a protein, potentially numbering more than 10,000. These variants are compared to the “original” wild-type protein to assess the effects of each mutation, though attempting to examine the compounding effects of multiple amino acid mutations and variants is difficult. DMS can provide insight on alteration effects on proteins and has historically been utilized to infer protein structure, function, and interactions.
We examined the realm of research of DMS, PFP, and PSP using research clusters (RCs) in CSET’s Map of Science.
Deep mutational scanning RC
We found one RC when searching for clusters using “deep mutational scanning” as a keyword.1 According to available data,2 the United States publishes the most papers in this RC, which includes less than two percent AI-related papers.
RC 4956
RC 4956, which falls in the broad subject area of biology, also focuses on computer science and chemistry, specifically zeroing in on genetics, computational biology, machine learning, protein structures, and virology – all important components to PSP. Nearly three-quarters of papers in this RC report a funder. Of those, 73 percent are government organizations and 25 percent are non-profit organizations, while the remainder are academic or industry organizations. Five percent of papers have a Chinese author, while 58 percent of papers have an author affiliated with the United States. The number of papers in this RC grew 37 percent in the last year. Below, we highlight the RC’s “core papers,” which are individual papers that are most highly connected to the other papers within the cluster through shared citations.
RC 4956 core papers
- Local fitness landscape of the green fluorescent protein, 2016, Nature
- The dynamics of molecular evolution over 60,000 generations, 2017, Nature
- Epistasis in protein evolution, 2016, Protein Science
- Tempo and mode of genome evolution in a 50,000-generation experiment, 2016, Nature
- Pairwise and higher-order genetic interactions during the evolution of a tRNA, 2018, Nature
Protein structure prediction and protein folding problem RCs
We retrieved six RCs with either “protein structure prediction” or “protein folding problem” as keywords, using the same process as above. Unlike “DMS,” “protein folding” and “protein structure” are specifically mentioned in our subject field classification — see Microsoft Academic Graph (MAG). By searching these subject fields, we garnered 38 more RCs. In total, we retrieved 44 “protein structure” or “protein folding” RCs.
Of these, 26 are led by the United States and 10 are led by China, in terms of publications. Twenty fall in the broad subject area of biology, nine in computer science, seven in physics, six in chemistry, and one in each of mathematics and materials science.
Figure 1. Number of papers from top publishing countries in 44 protein-folding-related RCs
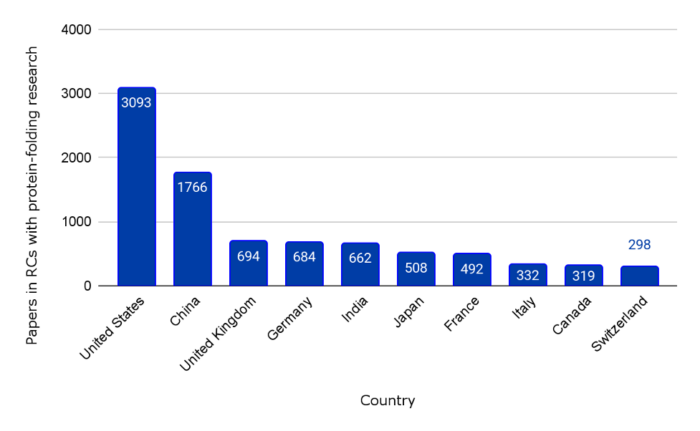
When taking all 44 of the identified RCs together, authors affiliated with the United States publish the most papers, followed by authors affiliated with China.
Of the 44 PFP/PSP RCs, one (RC 66000) qualifies as an advanced AI RC, defined as having more than 75 percent AI-related papers. RC 66000 has 77 percent AI-related papers, and 5 percent computer vision (CV) papers.
Of the other 43 PFP/PSP RCs, four have 25-50 percent AI-related papers, seven have 10-25 percent AI papers, and seven 5-10 percent AI papers. The remainder have less than 5 percent AI papers. Below, we examine the two RCs with the highest concentrations of AI papers, and the RC with the highest number of average citations.
RC 66000
RC 66000 is led by China, with 38 percent of its papers having a Chinese author affiliation. This RC focuses on computer science with secondary foci in mathematics and biology. In addition to having over 77 percent AI-related papers, this RC also has over 5 percent computer vision-related papers. The number of papers in RC 66000 grew 71 percent in the last year.
RC 66000 core papers
- Protein fold recognition based on sparse representation based classification, 2017, Artificial Intelligence in Medicine
- An Enhanced Protein Fold Recognition for Low Similarity Datasets Using Convolutional and Skip-Gram Features With Deep Neural Network, 2020, IEEE Transactions on NanoBioscience
- ProFold: Protein Fold Classification with Additional Structural Features and a Novel Ensemble Classifier, 2016, BioMed Research International
- Relevance of Machine Learning Techniques and Various Protein Features in Protein Fold Classification: A Review, 2019, Current Bioinformatics
- Enhanced Artificial Neural Network for Protein Fold Recognition and Structural Class Prediction, 2018, Gene Reports
RC 6554
The PFP/PSP cluster with the second-highest concentration of AI-related papers (nearly 50 percent), RC 6554, is a computer science cluster also led by China, with the United States in second. RC 6554 focuses on data mining, machine learning, biological systems, and pattern recognition. The number of papers in this RC grew by 79 percent over the last year.
RC 6554 core papers
- MUFOLD‐SS: New deep inception‐inside‐inception networks for protein secondary structure prediction, 2018, Proteins Structure Function and Bioinformatics
- Sixty-five years of the long march in protein secondary structure prediction: the final stretch?, 2016, Briefings in Bioinformatics
- Capturing non-local interactions by long short-term memory bidirectional recurrent neural networks for improving prediction of protein secondary structure, backbone angles, contact numbers and solvent accessibility, 2017, Bioinformatics
- Prediction of 8-state protein secondary structures by a novel deep learning architecture, 2018, BMC Bioinformatics
- Evolution of fold switching in a metamorphic protein, 2020, Science
RC 20538
RC 20538 has the highest number of average citations from the identified PFP/RCs, with over 19 average citations per paper. It also has the fourth-highest concentration of AI-related papers at 32 percent. The United States is the most common country of affiliation for authors, with 47 percent of papers with a United States-affiliated author. This RC focuses on algorithms, data mining, and computational biology, in addition to protein structures.
RC 20538 core papers
- Accurate De Novo Prediction of Protein Contact Map by Ultra-Deep Learning Model, 2016, bioRxiv
- Distance-Based Protein Folding Powered by Deep Learning, 2019, Research in Computational Molecular Biology
- Deducing high-accuracy protein contact-maps from a triplet of coevolutionary matrices through deep residual convolutional networks, 2020, bioRxiv
- DeepMSA: constructing deep multiple sequence alignment to improve contact prediction and fold-recognition for distant-homology proteins, 2019, Bioinformatics
- Protein structure determination using metagenome sequence data, 2017, Science
Be on the lookout later this month for our final topic-based Map of Science data snapshot of the year, and find the earlier snapshots, including examinations of AI, NLP, and robotics RCs, below.
- Here, we searched for either author subject labels or top extracted phrases (words that frequently came up in papers within a cluster).
- Note that a single paper can be affiliated with multiple countries. For example, if one paper has authors from the United States, China, and South Korea, then that would count as one paper for each of those three countries.