In our previous data snapshot, Keyword Cascade Plots, we demonstrated how we can visualize research clusters of interest and their connections via citation links using CSET’s Map of Science and a set of keywords. Here, we highlight how to use the keyword cascade plot as a data selection tool to identify research clusters that are relevant to an analytical task. We will continue our exploration of deep learning research from the previous snapshot, displayed below in Figure 1, and show how the keyword cascade plot can help explore security-relevant applications of deep learning. Note that the analysis presented here is done on the underlying merged corpus data, and cannot be replicated directly in the Map of Science interface.
Figure 1. Deep Learning Keyword Cascade Plot
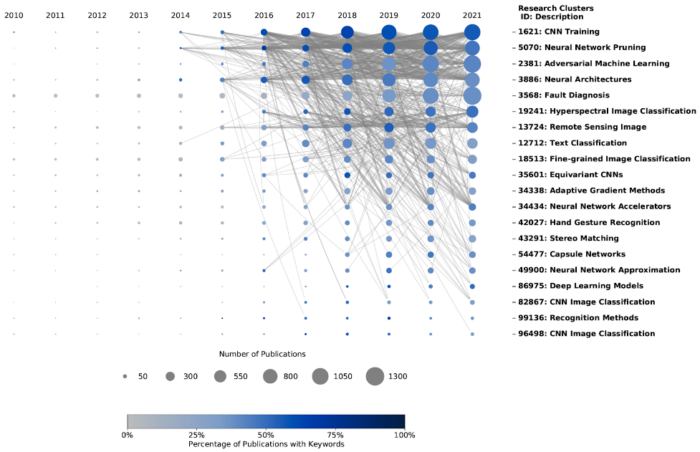
The Map of Science includes more than 100,000 research clusters, which may seem overwhelming when looking for a specific area of research or trying to explore applications of that research. The keyword cascade plot enables analysts to pinpoint specific research clusters within an area of interest to explore with narrower scope.
Each research cluster in the Map of Science has aggregated metadata describing the member publications using features such as key concepts and percentage of AI-related publications. The key concepts field is generated using a phrase extraction algorithm and provides the top five concepts mentioned across a research cluster’s member publications. We use the leading key concept as an automatic text label to describe the research clusters displayed in the keyword cascade plot (Figure 1 shows the key concept label immediately following the cluster’s ID number).
In this example, we will use the key concepts field to find areas where deep learning is related to an application important to security. We select research clusters to include in our analysis of security-relevant applications of deep learning. Reviewing the key concept labels in Figure 1, two research clusters stand out as clearly security-relevant based on their leading key concept.
- Remote Sensing Image (cluster 13724)
- Text Classification (cluster 12712)
Remote Sensing Image
Research cluster 13724 contains 927 publications that mention the terms “deep learning” or “convolutional neural network” in their titles or abstracts (42 percent of all cluster publications). Figure 2 displays the keyword cascade plot with only the links to cluster 13724 shown. We see the deep learning keyword publications in this cluster cite other keyword publications from eight other research clusters, and maintain the citation links over time.
Figure 2. Remote Sensing Image Cluster Links
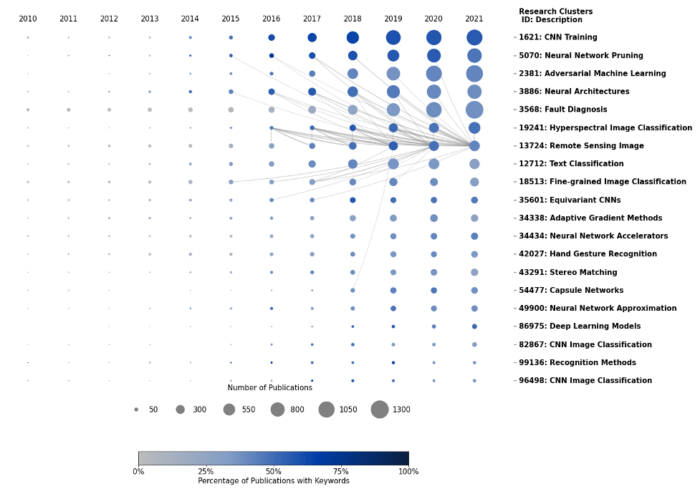
In order to understand these links, we investigate the 520 unique publications with citation links between the remote sensing image cluster and the eight linked research clusters. The research cluster with the most links to our remote sensing image cluster is hyperspectral image classification (cluster 19241), with 179 publications cited by cluster 13724. In Table 1, we list the top five most cited publications in cluster 19241 with links to cluster 13724.
Table 1. Top Five Most Cited Publications in Research Cluster 19241
We can see that the primary use of deep learning in this research cluster is classification of hyperspectral data, leading to an improvement in the speed and automation of a previously labor intensive task in the use of remote sensing imagery. This example may seem obvious, however the technique used can be a powerful one when exploring emerging or more obscure areas of science and can improve our ability to see the application of science across domains.
Text Classification
Research cluster 12712 contains 894 publications that mention the terms “deep learning” or “convolutional neural network” in their titles or abstracts (35 percent of all cluster publications). As shown in Figure 3, the text classification cluster has notably fewer deep learning citation links to other clusters; it is only linked to three other research clusters. Additionally, these citation links are not consistent over time.
Figure 3. Text Classification Cluster Links
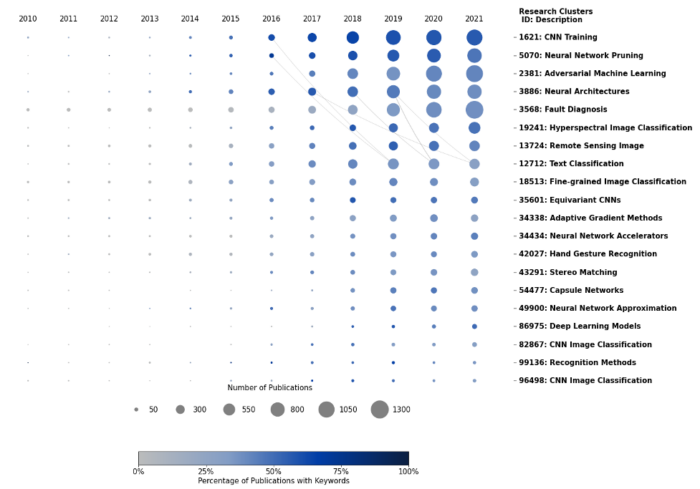
The few citation links between the text classification cluster and the other three research clusters represent 158 unique research publications. The research cluster with the most links to the text classification cluster is on neural architectures (cluster 3886), with 53 unique publications cited by deep learning publications in the text classification cluster. In Table 2, we list the top five most cited publications in cluster 3886 with links to cluster 12712.
Table 2. Top Five Most Cited Publications in Research Cluster 3886
Here, we see that the top five papers are more general research publications in the deep learning and classification space, as the cluster is focused on neural architectures. Again, the possible application of deep learning for text classification is much easier to discern by looking at citation linkages within a specific area of research than by only analyzing publications that match a keyword list.
In the next data snapshot, we will capitalize on the ability to show directionality using this approach—specifically, how we can see what papers in a cluster reference data or information from an older paper (i.e. importing information) and what papers are being cited by newer papers (i.e. exporting information). This will allow us to further prioritize research clusters of interest and show how they are affected by, and are affecting, other research across domains.