In a previous snapshot, Defining AI-Supported Research Clusters, we described how we identify the varying levels of artificial intelligence relevance in the research clusters (RCs) in our Map of Science. Here, we define how we distinguish between miscellaneous AI/machine learning (ML) research and three related research areas: computer vision (CV), natural language processing (NLP), and robotics (RO).
We leverage the same SciBERT classifier used to identify a publication as AI-relevant as we use to label research publications as CV-, NLP-, or RO-related. Because four separate classifiers were trained for each research area (AI, CV, NLP, and RO), the research topic labeling is not mutually exclusive. This means that a single publication can be assigned more than one of the topic labels. These four topic labels can be used to differentiate between RCs that focus on general AI research and three common fields of research related to AI.
The research topic labels assigned to individual publications are aggregated at the RC level to produce a percentage of AI-, CV-, NLP-, and RO-related publications in an RC. Using those percentages, we assign a categorical label to each RC that indicates the most common AI-related topic in that RC, as displayed in Figure 1. Since we are interested in AI-related research, we assign RCs with 10-24 percent AI publications with the label of “cross-disciplinary AI/ML” and then only assign CV, NLP, and RO labels to RCs that have at least 25 percent AI publications.1 For those RCs, we check the percentage of papers in the RC that are CV-, NLP-, or RO-related. If none of these percentages is at least 25 percent, we label that cluster “miscellaneous AI/ML.” If at least one of the CV, NLP, or RO percentages is 25 percent or higher, we label that cluster as CV-, NLP-, or RO-related depending on which of the three has the highest percentage of papers in the cluster.
Figure 1. Diagram of AI-related research topic label assignment to RCs
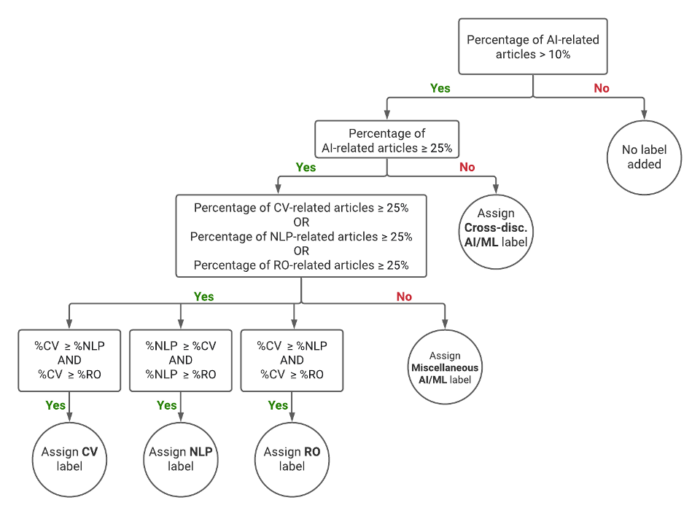
This labeling process results in 7,397 RCs assigned an AI-related topic (as of February 2021). Figure 2 displays the percentages of RCs in each AI-related topic. Almost half of these RCs are labelled as cross-disciplinary AI/ML. The miscellaneous AI/ML category represents the largest portion of the 7,397 RCs at 28 percent, while CV, RO, and NLP follow with 15 percent, 7 percent, and 5 percent, respectively.
Figure 2. Breakdown of RCs with more than 10 percent AI-related papers by AI-related topic
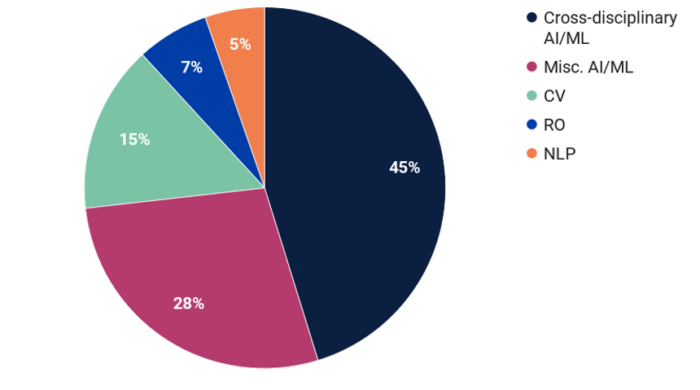
Next, we analyze the breakdown of RCs by their broad research area and AI-related topic to analyze the varying uses of AI across all of science. We find that for all broad areas of research, the cross-disciplinary AI/ML category still leads, but in computer science, miscellaneous AI/ML makes up a comparable proportion of RCs. In humanities and social science RCs, NLP leads CV and RO. Chemistry is the only broad research area that contains only cross-disciplinary AI/ML RCs.
Figure 3. Percentages of RCs by broad research area and AI-related topic
To provide an example of a RC with a CV, NLP, or RO label, we filter for forecasted extreme growth2 and then sort by percentage of AI-related papers in descending order. A resulting RC is 9079, which falls under computer science and is focused on human-computer interaction for visual navigation. Labeled as robotics, RC 9079 has 53 percent RO-related papers, but also has 52 percent CV-related papers, and 23 percent NLP-related papers. This showcases how these research topic indicators are not exclusive and are able to preserve cross-disciplinary research.
Similar to our analysis of the location of AI-related papers across the Map of Science,3 in our next snapshots, we will provide more details on the distribution of CV-, NLP-, and RO-related RCs.
In August 2021, CSET updated the Map of Science, linking more data to the research clusters and implementing a more stable clustering method. With this update, research clusters were assigned new IDs, so the cluster IDs reported in this Snapshot will not match IDs in the current Map of Science user interface. If you are interested in knowing which clusters in the updated Map are most similar to those reported here, or have general questions about our methodology or want to discuss this research, you can email cset@georgetown.edu.
Download Related Data Brief
Comparing the United States’ and China’s Leading Roles in the Landscape of Science- Autumn Toney, “Locating AI Research in the Map of Science” (Center for Security and Emerging Technology, July 2021).
- Autumn Toney, “Measuring AI RC Growth” (Center for Security and Emerging Technology, July 2021).
- Autumn Toney, “Locating AI Research in the Map of Science” (Center for Security and Emerging Technology, July 2021).