Exploring individual research clusters in CSET’s Map of Science can provide interesting insights (like Exploring Clusters of Research in Three Areas of AI Safety and Comparing the United States’ and China’s Leading Roles in the Landscape of Science). With a new method, described in our last two data snapshots (Keyword Cascade Plots and Diving into Deep Learning with Keyword Cascade Plots), we can also analyze the relationship between research clusters using their citation linkages.
A valuable characteristic of citation linkages is their directionality – a publication can cite other papers and a publication can be cited by other papers. We consider these citation relationships analogous to sourcing, authors import information from other papers to build on prior work, and they export their information to papers published at a later date. For example, in Figure 1 we display how the publication “Learning Transferable Architecture for Scalable Image Recognition”1 (middle paper in figure 1) “imported” information from a previously published paper and “exported” information that was used in support of research from a subsequently published paper.
Figure 1. Paper-level Import and Export Citation Relationships
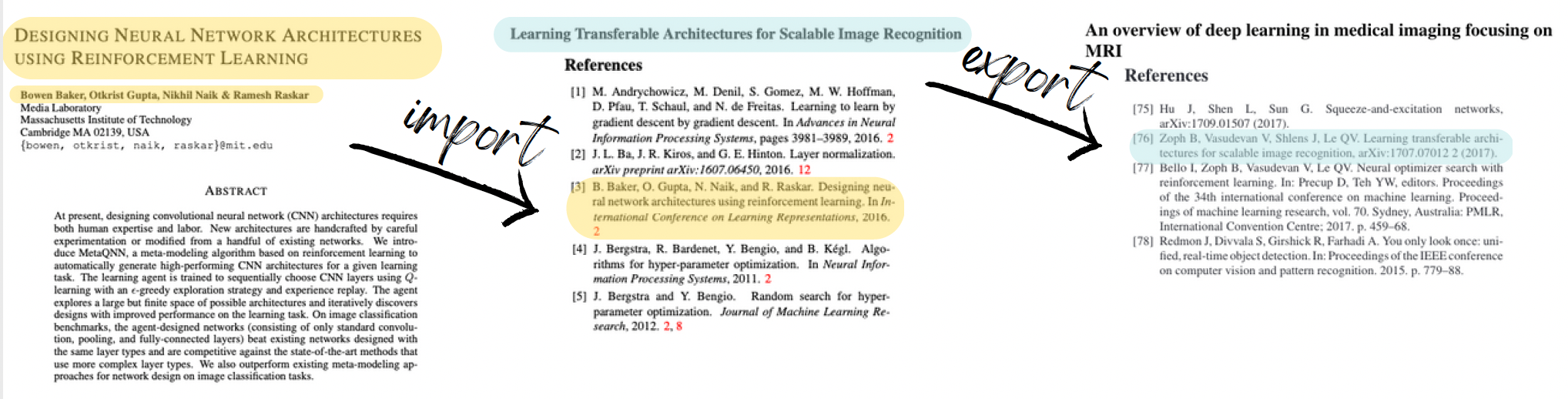
In this example, we see that the imported paper (Designing Neural Network Architectures Using Reinforcement Learning) is more general (basic research), as it focuses on neural network architecture design using a specific mode of learning. The paper of interest is less general, as its architecture was designed for image recognition tasks. The ideas from that paper were then exported to another paper (an overview of deep learning in medical imaging, with a focus on MRI), which is even less general (applied research) and is specifically reviewing deep learning techniques for MRI medical imaging. This example illustrates how the directionality of citation linkages can be used to identify translational science.
This import and export dynamic can be applied in aggregate to research clusters, to construct translational science pathways in the Map of Science.2
As shown in Figure 2, we can take a research cluster of interest (denoted in blue) and track its citation relationships to other clusters using member publications.
Figure 2. Cluster-level Import and Export Citation Relationships
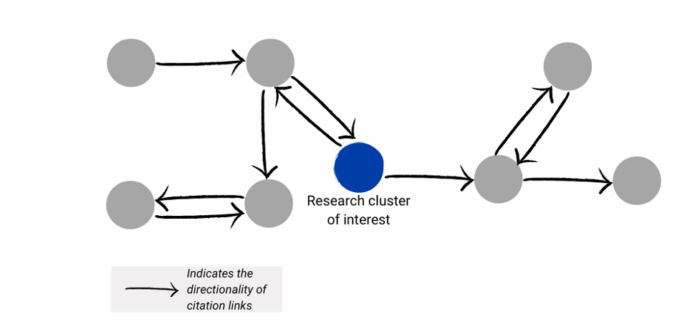
In this way, we are able to follow the paths of research imports or exports and track the development of research from cluster to cluster from an initial research cluster of interest. Using keyword search and techniques to select a cluster of interest (as we did in the Keyword Cascade Plots: a Visual Tool for Research Cluster Selection and Diving into Deep Learning with Keyword Cascade Plots snapshots to find clusters with deep learning research), we can choose a representative cluster for our task and investigate its import and export characteristics. Analyzing that cluster’s import, export, and member publication counts over time highlights the flow and interdependence of research. This highlights cases where, and the ways in which, more basic research communities produce work that flows to use in more applied settings. Below we show two example research clusters from our Deep Learning keyword results. Note that the analysis presented here is done on the underlying merged corpus data, and cannot be replicated directly in the Map of Science interface.
In Figure 3, we display the publication counts over time for research cluster 3886 (Neural Architectures). We find that citations of this cluster greatly increased (exports) in 2017, far surpassing the number of total member publications over time. If we analyze only the growth of the research cluster in isolation, ignoring the growth of its exports or use of the knowledge created by that research, we might miss valuable signals for the shift from research to application.
Figure 3. Research Cluster 3886 Import, Export, and Cluster Publication Counts Over Time (2016-2021)
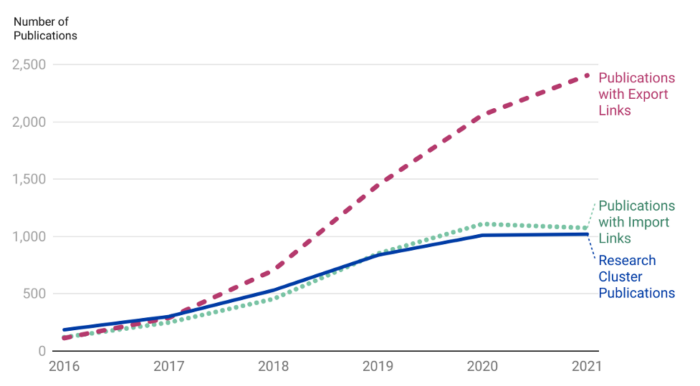
In Figure 4, we display the publication counts over time for research cluster 19241 (Hyperspectral Image Classification). This example shows a cluster in which the number of import link publications is constantly greater than the total number of member publications and export link publications over time. This cluster has more papers that import knowledge from other clusters but fewer papers being exported to other clusters, though the number of export publications is increasing over time, in line with cluster publications and import publications.
Figure 4. Research Cluster 19241 Import, Export, and Cluster Publication Counts Over Time (2016-2021)
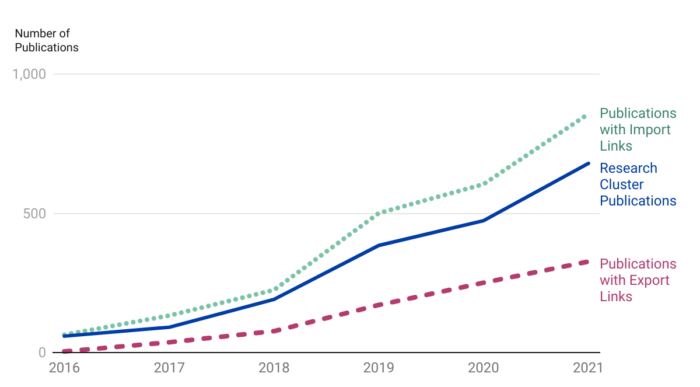
Policy questions often revolve around deciding which areas of emerging science are progressing most quickly and need the most attention, both through the lens of investment and evolution of the threat. This approach indicates that while research cluster 19421 is still growing quite quickly (see Figure 4), the uptake of that research is not growing as rapidly. This is research to watch—the research itself is moving, but uptake and usage in other areas is still slow. On the other hand, research cluster 3886 indicates an uptake of that research (see Figure 3). And this, in turn, suggests it is time to look at the clusters to which it is exporting and shift our focus from the science to the growth of the application space. It also suggests that instead of simply focusing on experts within the specific research area, policymakers might also want to leverage experts in domains that are applying the research in order to understand its progress and potential impact.
- Zoph, Barret, Vijay Vasudevan, Jonathon Shlens, and Quoc V. Le. “Learning transferable architectures for scalable image recognition.” In Proceedings of the IEEE conference on computer vision and pattern recognition, pp. 8697-8710. 2018.
- Smith, Caleb, Klavans, Richard, and Boyack, Kevin. “A Bibliometric Solution to the Problem of Translational science.” International Conference on Science, Technology, and International Indicators (2022)