To understand how artificial intelligence (AI) and machine learning affect areas of research across all of science, we explore CSET’s merged scientific publication dataset1 (containing roughly 90 percent of the world’s scientific literature) via an automated structuring process that groups publications into research clusters.2 RCs are groupings of scientific publications based on direct citation links, meaning that publications in a given RC cite one another more than they cite publications in other RCs. They are organized on the map of science by their citation relationships—RCs with stronger citation links are spaced closer together.
Figure 1. Map of Science
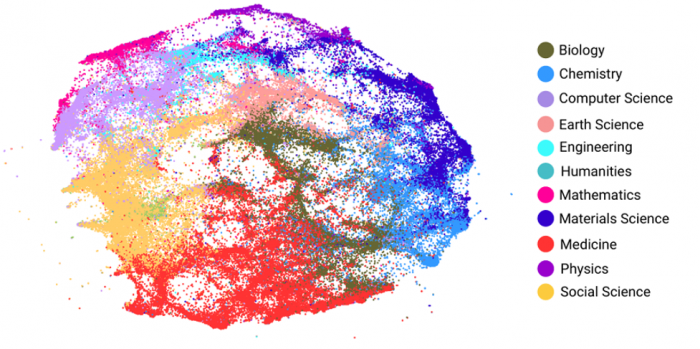
As displayed in Figure 1, we can assign RCs, represented as individual dots on the map of science, to a research area. Here we use 11 broad areas of research: Biology, Chemistry, Computer Science, Earth Science, Engineering, Humanities, Materials Science, Mathematics, Medicine, Physics, and Social Science.
We can determine the percentage of AI-related papers, CV-related papers, NLP-related papers, and RO-related papers in a given RC. This allows us to identify RCs with a range of AI applications.
We can also identify RCs that are AI-related. To do this, we compute the percentage of papers within a given cluster that have been classified as AI-related using a SciBERT model trained on arXiv publications. Publications with at least one label of cs.AI, cs.LG, cs.MA, or stat.ML were classified as AI. 3 All papers published in English from 2010 through present day are assigned a label of AI-related (or not AI-related) using Dunham et al.’s classifier, and papers published in Chinese are labeled as AI (or not) via regular expression queries.4 The classifier also assigns three additional labels to a paper: computer vision (CV), natural language processing (NLP) and robotics (RO). The classifier is not limited to one label in its assignment (i.e., an article can be labeled as computer vision and robotics).
Using this method to classify individual papers, we can determine the percentage of AI-related papers, CV-related papers, NLP-related papers, and RO-related papers in a given RC. This allows us to identify RCs with a range of AI applications. Figure 2 highlights RCs with 10 percent or more AI-related papers in the map of science. Future work will analyze how to use these AI-related percentages meaningfully when exploring the RCs.
Figure 2. RCs with 10 Percent or more AI-related papers highlighted in the map of science
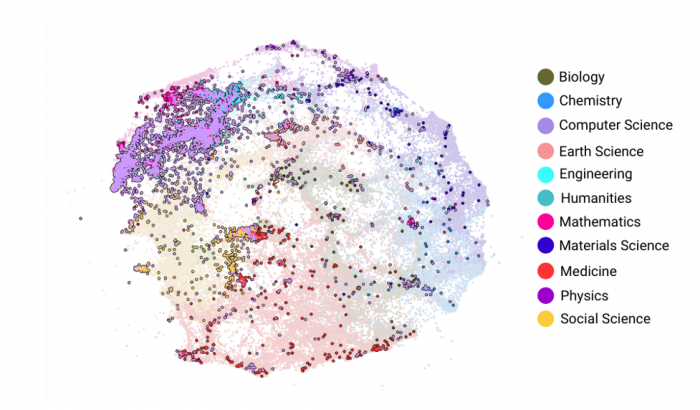
To ensure confidence in any analysis focusing on AI RCs, we suggest filtering RCs based on two criteria: 1) RCs where 50 percent or more of the papers in that cluster were successfully assigned an AI (or not AI) label and 2) RCs with an “age” of 20 years or less. In effect, this results in a sample of RCs with a reliable measure of AI-relevance that have existed for the past 20 years.
If you have questions about our methodology or want to discuss this research, you can reach us at cset@georgetown.edu.
Download Related Data Brief
Comparing the United States’ and China’s Leading Roles in the Landscape of Science- This dataset contains Web of Science, Digital Science, Microsoft Academic Graph, and the Chinese National Knowledge Infrastructure.
- Rahkovsky et al. “AI Research Funding Portfolios and Extreme Growth” and Frontiers in Research Metrics and Analytics. (2021): 6.
- James Dunham, Jennifer Melot, and Dewey Murdick (2020) Identifying the Development and Application of Artificial Intelligence in Scientific Text.
- Rahkovsky et al. “AI Research Funding Portfolios and Extreme Growth”and Frontiers in Research Metrics and Analytics. (2021): 6.